
Monitoring atmospheric NO₂ allows prediction of whether containment is effective against a pandemic

A model based on machine learning techniques and satellite data can predict whether reducing economic activity reduces pandemic infections. The aim is to better fine-tune the timing and degree of containment measures.
An international study with the participation of Josep Peñuelas, CREAF and CSIC researcher, and Jordi Sardans, CREAF researcher, has developed a model to predict the effectiveness of containment to curb epidemics such as Covid19, based on satellite data obtained from nitrogen dioxide pollution (NO₂). The model developed is able to predict how infections accelerate when containment measures are lifted. The information therefore allows optimising the timing and intensity of non-pharmaceutical interventions, and improving the effectiveness of control of Covid19 and pandemics in general.
The scientific team has applied machine learning techniques to monitor how economic activity is reduced by monitoring nitrogen dioxide levels in the atmosphere in near real time.
The scientific team has applied machine learning techniques that make it possible to monitor how economic activity is reduced by controlling the levels of nitrogen dioxide in the atmosphere in almost real time. Researcher Josep Peñuelas explains that "the model significantly improves the predictions used so far by the World Health Organisation and other organisations, both governmental and non-governmental".
The study was published in the journal PNAS and involved specialists in air pollution, economics, epidemiology, data analysis and artificial intelligence from around 20 research centres.
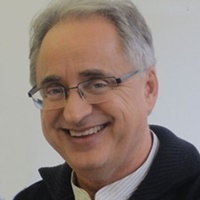
"The model we have developed significantly improves on the predictions used so far by the World Health Organisation and other organisations, both governmental and non-governmental"
JOSEP PEÑUELAS, CREAF and CSIC researcher.
“As we have seen in the work, about one million Covid19 cases per day could have been avoided in winter 2020-2021 if the time and levels of containment restriction had been optimised," says Rong Wang, a scientist at Fudan University (China) and coordinator of the research, which was funded by the Agency for Management of University and Research Grants (AGAUR).
Nitrogen and economic activity
To train the model, NO₂ levels observed by satellites in the weeks of confinement after the Covid19 outbreak in 2020 were compared with those of the 2016-2019 period in the same areas. The observations cover 211 geographical areas, of which 31 are provinces in China, 51 are US states and 129 are countries in Europe, Asia, the Middle East, Africa and Latin America. These data have been correlated with the numbers of infections in each of these areas in the weeks of containment and subsequent weeks when the measures were lifted.
The calculations have taken into account meteorological, environmental and social variables that can influence both NO₂ levels and the spread of infections, and which are unrelated to economic activity
In addition, predictions have been adjusted to take into account meteorological, environmental and social variables that can influence both NO₂ levels and the dispersion of infections, and which are not related to economic activity. The resulting model can predict the slowdown in infectiousness in the 211 areas from the NO₂ observations and the 10 most determinant environmental and socio-economic indicators. The model also allows identifying other possible outcomes depending on the measures implemented.
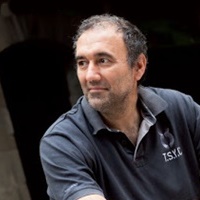
"This study provides a quantitative assessment of the effectiveness and timing of non-pharmacological interventions such as containment to contain epidemics such as Covid19"
JORDI SARDANS, researcher at CREAF.
"We knew that non-pharmacological measures such as confinement are effective in containing epidemics such as Covid19, but we still lacked a quantitative assessment of the effectiveness and timing of these interventions in different regions of the world," concludes the CREAF researcher Jordi Sardans.
Reference article:
Xiaofan Xing et al. 2021. Predicting the effect of confinement on the COVID-19 spread using machine learning enriched with satellite air pollution observations. PNAS. DOI: 10.1073/pnas.2109098118
Related news

A set of proposals for political action in the Mediterranean against the climate crisis is presented at COP29

